The Role of Generative AI Cybersecurity in Preventing Cyber Threats
Cyberattacks aren’t slowing down. In early 2024, reports showed over 2,000 breaches every single day. That’s about one every 39 seconds. And they’re not just hitting banks and governments. Retailers, hospitals, even small startups are targets. The stakes? Around $4.45 million per breach. Criminals are using AI to move faster. It’s not guesswork anymore. It’s scripts, automation, and synthetic bait. That same tech is now being used to fight back. Generative AI cybersecurity is flipping the script. It’s changing how we protect systems, detect threats, and respond to attacks. It learns, predicts, blocks. And it does it in real time.
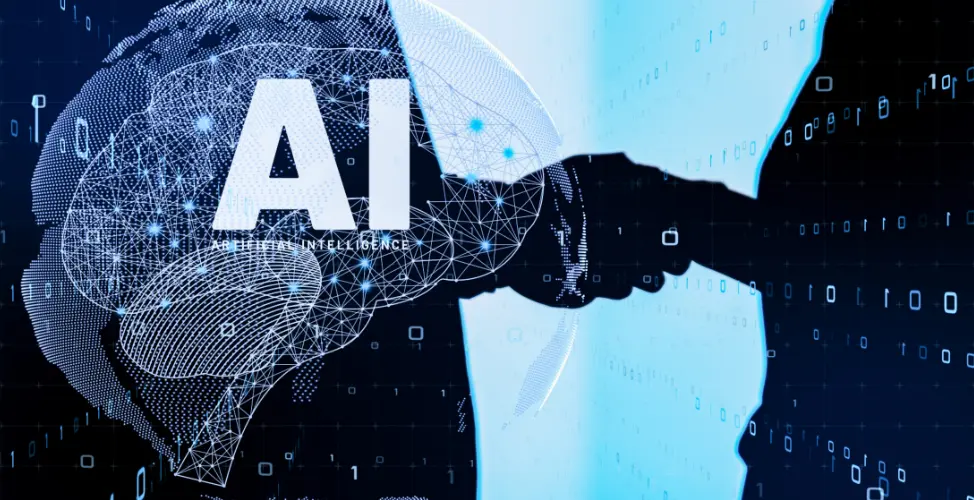
So how does this approach actually work and how do you use it without getting burned? Let’s break it down in this blog post!
Understanding Generative AI Cybersecurity
What is Generative AI Cybersecurity?
Generative AI cybersecurity is the practice of using AI models, especially ones that can generate new content, to boost digital defenses. Conventional security checks often rely on known signatures or patterns. In contrast, generative AI can model fresh threat strategies and figure out the best methods to detect or repel them. Think of it as having ‘virtual defenders’ that learn from historical data but aren’t bound by it.
This field focuses on training AI systems using real-world attack logs, network anomalies, and coded exploits. With the power of cloud computing AI, these models can analyze vast datasets at scale, learning to detect and block suspicious activity in real time—often before a threat can escalate. Unlike static security tools, cloud-based AI continuously evolves, updating itself daily to recognize new attack patterns. If cybercriminals tweak a piece of malware, the AI can quickly detect the change and respond, offering dynamic and proactive protection across cloud environments.
Features and Capabilities of Generative AI Cybersecurity
Here’s where generative AI really flexes its muscles in cybersecurity:
- Adaptive Threat Modeling: Rather than depending on rules set in stone, generative AI algorithms evolve based on new attacks. This approach lessens false positives.
- Synthetic Data Generation: Generative AI can create entire data sets that reflect real network traffic or user actions. This helps testers and security teams get ample data to prepare for threats without risking sensitive info.
- Early Intrusion Alarms: By learning typical user or system behavior, generative AI spots weird patterns right away. That might be a hidden script or abrupt data exfiltration attempt.
- Automated Patching Suggestions: Instead of waiting for patch cycles, it can propose immediate fixes, capturing vulnerabilities quickly.
- Real-Time Response: AI-based systems can take action on the fly, quarantining suspicious apps or forcing password resets when it observes high-risk signals.
Current Trends in Cyber Threats and AI Integration
Cyber threats have gotten more devious. Attackers are harnessing AI to craft highly customized phishing emails or produce convincing deepfakes. They sometimes rely on ‘bot armies’ to infiltrate systems or brute-force login pages. According to the World Economic Forum’s Global Cybersecurity Outlook 2025, 72% of organizations reported an increase in cyber risks, with nearly half citing AI-driven threats as a top concern.
On the flip side, defenders have boosted capabilities by adopting AI-driven solutions. These solutions keep track of critical assets, watch user behavior, filter spam, and coordinate responses. More organizations also incorporate zero trust architecture, assuming no user or device is trustworthy by default. Generative AI cybersecurity extends zero trust further by analyzing context, not just user credentials.
Watch more: The Future of AI in Customer Experience: Trends and Innovations
How Generative AI is Revolutionizing Cybersecurity Defense
Enhanced Threat Detection and Analysis
At the heart of generative AI cybersecurity is advanced threat detection. Conventional antivirus software works by referencing known signatures of malicious files. This is great for run-of-the-mill viruses. But it might fail against new or obfuscated malware. Generative AI handles anomalies better. It ‘reads’ network flows in real time. It merges logs from multiple endpoints. When a user visits a site or opens an email containing unknown code, the system flags suspicious payloads and warns the SOC (Security Operations Center).
In 2024, the number of data compromises in the United States reached 3,158 cases, affecting over 1.35 billion individuals. Implementing generative AI can help reduce the time between an intrusion and its detection by your team.
Automated Incident Response and Mitigation
Time is key when a breach occurs. The more minutes you take to respond, the more data might be stolen. Traditional incident response demands multiple steps: detect, classify, prioritize, respond, and remediate.
Generative AI can automate many of these tasks at once. Once an alert is triggered, an AI-run system can isolate compromised devices, lock suspicious accounts, and start preliminary forensics. It can also craft a quick step-by-step plan for your security engineers.
With AI through the cloud, there’s no need to wait for manual responses to threats. Instead, AI engines can automatically block malicious IP addresses or deploy new firewall rules in real time. Major cloud providers already use this technology for real-time traffic shaping, especially when they detect potential DDoS attacks. This level of automation not only enhances security but also reduces the burden on IT teams, allowing human experts to focus on more complex and strategic tasks.
Proactive Threat Prediction and Vulnerability Analysis
One bright spot of generative AI is anticipating future problems. By analyzing volumes of historical threats, it recognizes patterns hinting at vulnerabilities. Suppose certain application frameworks or outdated server kernels appear in your environment. AI can rank them by exploit probability and recommend fixes. This forward-looking stance shrinks the ‘attack surface.’
Consider scenario testing: the AI simulates possible infiltration paths. It might show you how a social engineering attempt could escalate to admin privileges. That knowledge prompts stronger password policies or new multi-factor authentication steps. And because AI engines update daily with fresh data, they remain aware of newly discovered zero-day exploits posted in real time.
See more: Why a Strong Partner Strategy for AI is Key to Business Success
Best Practices and Countermeasures in Generative AI Cybersecurity
Data Protection and Privacy Measures
Data is the fuel driving AI’s success. But it must be safeguarded meticulously. If generative AI is fed poorly secured info, it’s only a matter of time before sensitive data seeps out or is manipulated by adversaries. One best practice is to use encryption in motion and at rest for all training and inference data. This ensures no unauthorized person can read what’s inside your data sets.
It’s also wise to store minimal logs. Limit who can see the raw data. For compliance with privacy rules like GDPR, anonymize personal info or remove it before training. A single slip can lead to major fines or brand damage. Data minimization is no longer just a suggestion; it’s crucial for AI-based security.
Mitigating Prompt Injection and Adversarial Attacks
Generative AI models, especially large language models (LLMs), might be tricked through prompt injection attacks. Hackers feed malicious prompts, forcing the AI to generate harmful text or reveal internal data. Meanwhile, adversarial attacks rely on special data inputs carefully designed to fool the AI, making it produce incorrect or dangerous outputs. We’ve seen criminals craft images that confuse image-recognition AI, or code snippets that bypass content filters.
To lower these risks, you can integrate filtering layers. One technique is restricting the AI’s responses to verified prompts only. Another is scanning user input for suspicious patterns. Above all, frequent model retraining with adversarial examples ensures the AI stays robust.
Establishing Robust Model Governance and Continuous Monitoring
Advanced security demands thorough oversight. That means putting together model governance guidelines that define who controls the model, how updates are tested, and who approves changes. If you let random staff tweak or re-train the AI, watch out for accidental exposures. Introduce version control so you can revert to older, stable versions. Keep a change log for every iteration.
Continuous monitoring means analyzing how the AI responds in production. If it starts giving weird or biased output, you catch it quickly. Some organizations run a second AI ‘observer’ to watch the main AI, which can reduce the likelihood of hidden tampering or logic drift. A regular cycle of auditing, checking logs, verifying user requests, adds extra security layers. While it sounds complicated, it saves bigger problems down the line.
How SmartOSC Supports Generative AI Cybersecurity Initiatives
The threat landscape keeps evolving, so do our defenses. Organizations often need experienced partners who can handle the entire digital environment, both the complexities of AI and the bigger technological puzzle. SmartOSC offers such forward-thinking services. With over 18 years of delivering secure, reliable technology solutions, SmartOSC bridges cybersecurity expertise with next-generation AI capabilities.
SmartOSC teams up with top-tier platforms such as AWS or Adobe, to build solutions that incorporate generative AI cybersecurity for predictive threat analysis, automated detection, and risk mitigation. From thorough audits to secure custom development and compliance efforts, SmartOSC ensures you can stay safe end to end.
In real-world engagements, SmartOSC has helped banks deploy advanced anomaly detection or e-retailers revamp entire workflows to fight fraud. Whether it’s defending vast commerce ecosystems or adopting a zero-trust structure, SmartOSC positions clients to get ahead of cyber threats without the dread of complicated systems. Our approach is simple yet proactive: see problems early, neutralize them quickly, and keep data safe across the board.
Conclusion
Cybercriminals’ attacks, including ransomware, phishing, and AI-driven hacks, keep getting sharper. Old defenses like blacklists and static rules just can’t keep up. Generative AI cybersecurity changes the game. It reads subtle threats, simulates attacks, and reacts fast, faster than most teams ever could. That’s how companies stay one step ahead. Smart businesses are already using it to avoid costly breaches and late-night chaos. If you’re ready to join them, SmartOSC’s cybersecurity solution can help. We’ve got the AI skills and cybersecurity muscle to lock things down.
Need a hand getting started? Contact us today. Let’s keep your business a few moves ahead of the bad guys.