What Does the Generative AI Ecosystem Refer To? Key Components & Insights
‘Generative AI’ is stirring a lot of buzz these days. Many see fresh chances for creativity, while others remain unsure of what it all really entails. What does the generative AI ecosystem refer to? This ‘ecosystem’ revolves around data, algorithms, and people who craft new ways to create digital content. Yet it is not just about code or software. It is about the combined efforts of hardware, data pipelines, and human skill sets to spark new products and services.
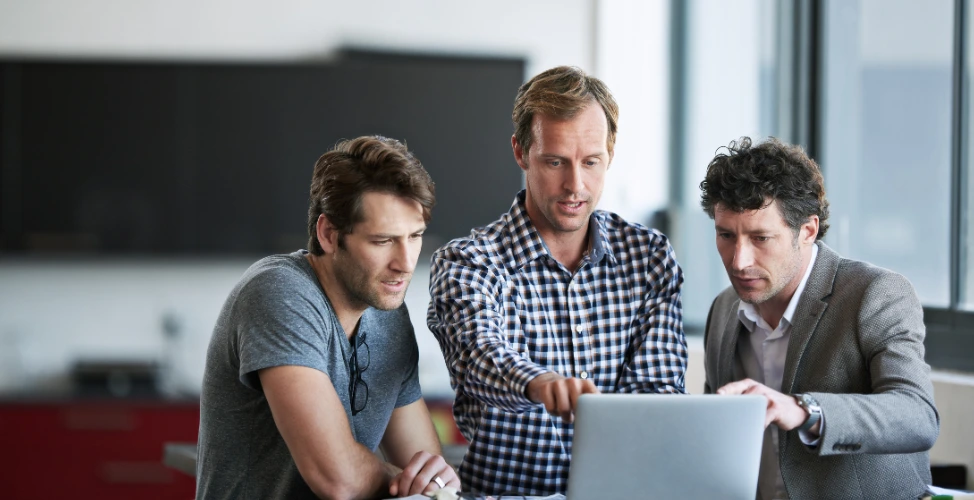
In this guide, we highlight the key parts that shape this ecosystem. We also explore key trends and share tips to prepare you for what lies ahead in the field.
Understanding the Generative AI Ecosystem
What does the generative AI ecosystem refer to? It encompasses far more than just text or digital art. This ecosystem includes tools and models that can generate music, write code, create video clips, and beyond. Each breakthrough within this space continues to reshape how we develop and interact with technology. To truly understand its impact, we need to start by breaking down the meaning and full scope of generative AI and the diverse capabilities it brings to various industries.
Definition and Scope
‘Generative AI’ covers software and models that create fresh output from patterns found in large sets of examples. This output might be an image, a conversation, or even code. The system looks at old data, then forms brand-new versions that can look eerily real or creative.
In earlier years, people viewed AI as a path to automate tasks. This model goes beyond that. It tries to craft new, original items. The line between human creativity and machine output is getting blurry, prompting more interest in this technology.
Evolution of Generative AI
Generative AI has deeper roots than some realize. Decades ago, folks tested basic language models that spat out random text. Those early attempts were very basic. Over time, hardware got faster, and research on neural networks made leaps.
Two big breakthroughs changed the scene. One was the ‘Transformer’ model, which excelled at understanding text structure in ways older models did not. The other was the rise of ‘diffusion’ methods and generative adversarial networks (GANs). These methods allowed for crisp images, lifelike voices, and more varied outputs.
Watch more: Why is AI So Popular Now? The Key Factors Behind Its Rise
Generative AI vs Traditional AI
Generative AI aims to ‘create’ while older AI methods mostly ‘detect’ or ‘classify.’ Traditional AI might tell you if an image is a cat or dog. It might create a new cat image from scratch.
Also, older AI often matches data to labels. This system tries to see patterns and produce new forms. This marks a shift in how we see AI, no longer just a tool for classifying data, but also a way to invent or dream up fresh ideas.
Key Components of the Generative AI Ecosystem
What does the generative AI ecosystem refer to in a structural sense? It involves distinct parts that, when combined, allow generative models to function smoothly. Each part has a role in fueling the entire cycle of creation.
Data Foundation
Data is the lifeblood of AI digital transformation. Without high-quality data, even the most advanced generative AI models can’t deliver reliable results. For example, a language model depends on rich text sources—like books, websites, or custom business data—while an image model requires well-labeled visual datasets. As companies undergo AI digital transformation, the quality and structure of their data become critical to unlocking meaningful, accurate, and impactful AI outcomes.
Data must be large enough, diverse, and free from major flaws. Also, the data should match your domain. If you train a language model mainly on news, you might not get the best results for medical topics.
Machine Learning Models
Generative models rely on advanced algorithms. Transformers are an example that made a big splash. Some large language models (LLMs) have billions of parameters. These parameters store what the model ‘learned’ from data.
Different tasks call for different model structures. GANs excel at producing crisp images but are not as good with text. Variational autoencoders can generate new samples of data but might not match the realism of newer approaches.
Computational Power
An AI model is only as quick or flexible as the hardware behind it. Training an LLM might take thousands of GPU hours. Memory capacity also matters, so large-scale computing is essential to handle complex tasks.
Public ‘cloud’ providers have stepped up by building solutions that help small or medium businesses rent large clusters of machines. This cuts the upfront costs. Tools from Cloud services might help you spin up or spin down computing resources as needed.
Feedback Loops
No model is perfect on the first try. Feedback loops let you refine it over and over. Users or testers might label outputs as correct or flawed. The system then ‘learns’ from that input, making adjustments.
For instance, if your generative model writes an odd or offensive sentence, you can tag it, so the next round of training addresses that. Over time, feedback loops ‘nudge’ the model closer to your ideal.
Technical Infrastructure
Building generative AI solutions requires more than just code. You need data storage, a pipeline for training, a pipeline for serving predictions, security measures, and fallback mechanisms in case your model fails.
Organizations often rely on version control, container orchestration, and load balancers. They might also depend on specialized systems to handle large data volumes and keep track of model versions.
Data Governance
Data governance is a formal way of making sure data is handled properly. It means you set rules on who can see, copy, or edit the data. You also track how data flows from the original source to your model.
Without data governance, you might violate privacy or lose track of which portion came from which set. Good data governance also helps you maintain consistent data quality.
Talent and Skills
At the heart of generative AI are human minds. Data scientists, machine learning experts, and domain specialists all play roles. A doctor might define the needed medical data for a health-based model. A data scientist then designs training steps, while a software engineer sets up the environment.
Beyond tech skills, you might need ‘communication gurus’ who can explain generative AI’s outputs to non-technical folks. Also, designers might craft user interfaces to present AI content in a friendly way.
See more: How Generative AI Architecture Powers Advanced AI Models
Insights into the Generative AI Ecosystem
What does the generative AI ecosystem refer to beyond the nuts and bolts? It also speaks to the broader implications for money, rules, industries, and upcoming directions.
Economic benefits
Generative AI for retail is set to transform how businesses operate, far beyond simple automation. From intelligent chatbots to on-demand content creation, it can streamline everything from customer service to marketing campaigns. By reducing time spent on repetitive tasks, generative AI helps retailers cut operational costs and redirect their teams toward more strategic, creative work—driving innovation and improving customer engagement at scale.
Yet there’s a big question: Will this tech cut entire job roles or spur brand-new ones? The truth likely sits somewhere in the middle. People might upskill or pivot to roles that involve guiding AI models.
Industry uptake
Many industries have shown interest in generative AI. A fashion brand might feed trend data into an AI that suggests new designs. An auto company might use it to create simulations or new part shapes. The ‘ecosystem’ thrives when each sector finds its path.
Healthcare, for instance, might harness these systems to interpret scans, while media might build AI-based story creators. The key is to figure out how these models can help without overstepping ethical or legal lines.
Ethical Factors
When a model learns from huge data sets, it can mimic biases or repeat untrue statements. That can lead to trouble. We must adopt guidelines to handle these issues, ensuring fair outcomes and mindful data usage.
Copyright also arises. If a generative model is trained on artworks, who owns the result? Different regions might have different rules. The ‘ecosystem’ thus must consider or create workable norms.
Future Trends
Right now, we see a swift rise in ‘agentic’ AI or self-directed models that not only generate text but also decide the next steps. Some call them ‘auto-GPT’ or ‘AI agents’ that might chain tasks together.
What does the generative AI ecosystem refer to? It includes not only current capabilities but also emerging technologies that could significantly expand its potential. For instance, quantum computing—though still years away—might one day supercharge generative AI performance. Another exciting frontier is multimodal models, which can understand and generate text, images, and audio simultaneously. These innovations could enable entirely new experiences, such as AI systems that watch a video and deliver real-time summaries, showing just how dynamic and evolving the generative AI ecosystem really is.
How SmartOSC Supports Generative AI-Driven Transformation
At SmartOSC, we see generative AI as more than a buzz term. Our approach connects strategic planning with real business needs. Our digital transformation services revolve around bridging the gap between new technology and daily operations.
We begin by looking at your main processes. We check how data is collected, stored, and labeled. Then we craft a plan to integrate generative AI solutions. The method is direct and business-focused.
We also realize no single approach fits all. Our teams combine domain knowledge, software engineering, and user design to mold a ‘tailored’ solution. That might mean building a text generation tool to produce product descriptions for eCommerce. Or it could be an AI model that sketches new ‘blueprints’ in the manufacturing field.
If you are looking for a partner to ‘jumpstart’ your AI efforts, consider SmartOSC. We bring a blend of strategy, ‘experience design,’ and technical execution. Also, we test thoroughly to confirm your system is reliable.
Conclusion
We have explored the major parts of this fascinating subject. What does the generative AI ecosystem refer to? In simple terms, it is the network of data, models, infrastructure, and human skill sets that come together to produce new digital creations. This ecosystem is not static. It is shaped by leaps in computing, feedback from users, and fresh uses that pop up every day.
Now is a prime time to learn about generative AI, try small projects, and plan bigger steps. As you do, keep in mind the ethical side and data requirements. If you want to ‘talk further’ or plan your next move, contact us or check out our ai digital transformation services. We stand ready to guide you toward real outcomes with generative AI.
Remember, this field is ‘just warming up.’ By staying curious and open, you can uncover new ways to grow or stand out. As you build and refine these AI-driven ideas, you shape a tech path that merges human creativity with smart systems. It is a chance to reimagine what is possible, one idea at a time.